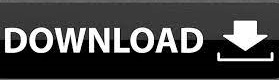
"Other important data attributes that play a role in selecting visualization primitives include functional dependencies among data variables, spacing between sampling points, cardinality of the data set, upper and lower bounds of values, units of measurement, coordinate system, scale, and continuity of data.".The number of components that specify a tensor depends on the dimensionality of the coordinate system and the order of the tensor. Tensors includes several scalar components that change in a particular way during transformation from one coordinate system to another. A Vector is generally a unified entity, consisting of several scalar-values. They require a number of scalar components, equal to the dimensions of a coordinate system. Vectors have in contrast of scalars, both, direction and magnitude. A scalar is simply defined as a single number. A scalar possesses a magnitude, but no directional information other than a sign. Quantitative data can be scalar, vector and tensor.
Quantitative data is more common than qualitative data in all scientific disciplines.A typical example of an ordinal data set is the names of the calendar month, January through December.
The ordering of the data does not reflect the magnitudes of the differences. Ordinal data types are rank ordered only. For example, the names of the discoverer, such as Gallileo, Columbus, Magellan, Marc'o Polo etc., form a nominal data set. Nominal data types are assemblages of symbolic names, typically unordered.
Qualitative data can be subdivide further-on into nominal and ordinal data types. You can divide scientific data into two groups: the qualitative and quantitative data. We will picture the marks and the composition rules especially. Under use with these five points, we will describe the individual concepts, declared by Ignatius and Senay. primitive visualization techniques,/li>. Ignatius and Senay classified the knowledge, which is necessary for scientific data visualization in five categories: First we will give an overview of the content of this side. We will explain the concept of marks later. Well, marks are the most primitive graphical objects, which constitute the graphics. If you have read the information about VISTA, an automated system by the authors, you have something read about marks. (This information is partially taken from the text, in which Vista, the automated visualization system is described.) This graphic by Andrew Abela from Extreme Presentations provides a good representation of different types charts that can be used to visualise data.Data, vocabulary, marks, composition rules and visual perception rulesĪn conceptual overview of the knowledge which is necessary for scientific data visualization Click on each to see examples and read advice on using and choosing that visualisation method. See the discussion under Report and Support Use for more information about how you may want to repackage a data visualisation for communication purposes.Įach main method below contains several visualisation possibilities. When communicating that data, however, the visualisation may need to be simplified and key areas may need emphasis in order to call the attention of readers and stakeholders. Visualisation, in this instance, eases data analysis. You may want to graph data during analysis to see, for example, spikes in website traffic related to your social media campaigns. The methods listed here can support both purposes of analysis and communication. Incorrect visualisation leads to confusion, errors, and abandonment among viewers. Experimentation with visualisations during analysis is okay, but when communicating a visualisation, use the graph types listed under the proper methods below. That said, sometimes graphing data with an inappropriate visualisation can lead to insights during analysis that would have remained hidden. Here we have adopted and modified the categorization system used by ManyEyes (archived link, IBM closed this service in 2015). The choice of what type of graph or visualisation to use depends greatly on the nature of the variables you have, such as relational, comparative, time-based, etc. Data visualisation is the process of representing data graphically in order to identify trends and patterns that would otherwise be unclear or difficult to discern.ĭata visualisation serves two purposes: to bring clarity during analysis and to communicate.